What Is Python, And Why Is It Awesome?
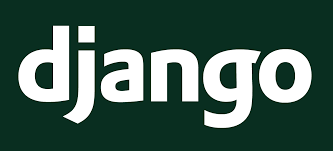
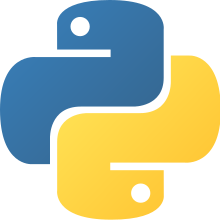
What is Python?
Python is a relatively intuitive, general-purpose programming language that allows users to do anything from analyzing data to building websites. In the context of data, it allows for complete flexibility and customizability when performing analyses. Here are some good resources that dive deeper into what Python is if you are interested:
https://www.coursera.org/articles/what-is-python-used-for-a-beginners-guide-to-using-python
https://www.geeksforgeeks.org/history-of-python/
Check out this nifty guide as well:
Why is it great?
When a client lets us choose our tools, we always choose Python. It’s the best in three main areas:
- Flexibility/Customizability. If an analysis is possible in a general sense, it’s possible to conduct that analysis in Python. In other words, in the data analytics world, if you can’t do it in Python, you can’t do it. Python offers countless libraries in data visualization, data processing, statistics, and more, allowing users to optimize any analysis for speed, storage, and accuracy.
- Scalability. Much of the world today remains stuck on Excel. That’s not completely a bad thing; Excel is a highly intuitive product that makes otherwise complex analyses quite straightforward for non-technical users. But where Excel falls well short of Python is in scaling. Excel files face a limit of just over one million rows per tab, which is not a limitation for Python; in today’s big data-backed data science world, one million rows of data is not that huge of a dataset, so Python therefore enables data scientists to complete more thorough analyses than Excel. And even if you can fit all of your data onto an Excel sheet, Excel’s performance starts to deteriorate much faster than Python when dealing with larger and larger datasets.
- Automation. If you want your dashboards, reports, and other analyses to update automatically, Python is the way to do that. Python specializes in compiling data from disparate sources (e.g., SQL databases, APIs, CSV files, and many more) into one place and inserting it into PowerBI, Tableau, or whatever other analytics tool you choose on a completely hands-free basis.
While we are familiar with R and other languages, and are happy to work in those languages as well, we choose Python for the reasons mentioned above. We think it’s the best of the best for data analytics and data science.
Applications of Python: API Integration
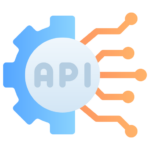
APIs (Application Programming Interfaces) are a tool that a developer can use to programmatically extract data from some other location in real time. For example, if you have data stored in Salesforce, Zoho, Monday, or other CRM, you can pull that data into a customized analysis in real time via an API using Python. Because of the increased level of automation that this method provides, it is seen as preferable to downloading data from the program into a CSV or Excel file and then repeating the analysis manually. While Python is not the only programming language that can be used to pull data from an API, it is advantageous to keep your end-to-end data process in one language, especially if the analysis of the data you’re pulling is to be completed in Python. Boxplot specializes in building fully-automated customized dashboards and reports for our clients using data stored in some third-party system; Python is always our preferred language for client projects along those lines.
It is also possible to insert data into a third-party storage system in real time using an API in addition to pulling data. This technique comes in handy when trying to ensure that multiple data storage systems don’t get out of sync; a Python-based program can automatically update data in some storage system A based on updates made in some other system B. Boxplot has also worked on many client projects involving this sort of work.
Applications of Python: Big Data
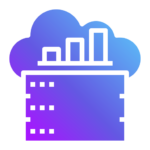
As aforementioned, Python does not face the same data volume limits that users run into with Excel. Python enables users to process even one million rows of data in a near-instantaneous manner on an average-quality computer. And if you anticipate utilizing a massive billion-row dataset, Python easily integrates with Spark, Hadoop, and other parallel computing frameworks. In short, if you’re going for anything big-data-related, Python is the way to do it.
Applications of Python: Machine Learning
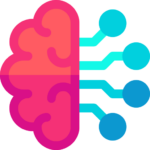
Machine Learning involves teaching a computer how to detect and extrapolate upon data without having to give it specific instructions on how to do so. Python is far and away the strongest programming language for machine learning, as numerous free-to-use machine learning libraries are available. These libraries include packages used in both supervised learning (i.e., when a computer tries to predict a set output) and unsupervised learning (i.e., when a computer creates abstract labels for categories of data observed in the dataset). Boxplot has completed numerous client projects in both supervised and unsupervised learning, and we always turn to Python as our go-to solution.