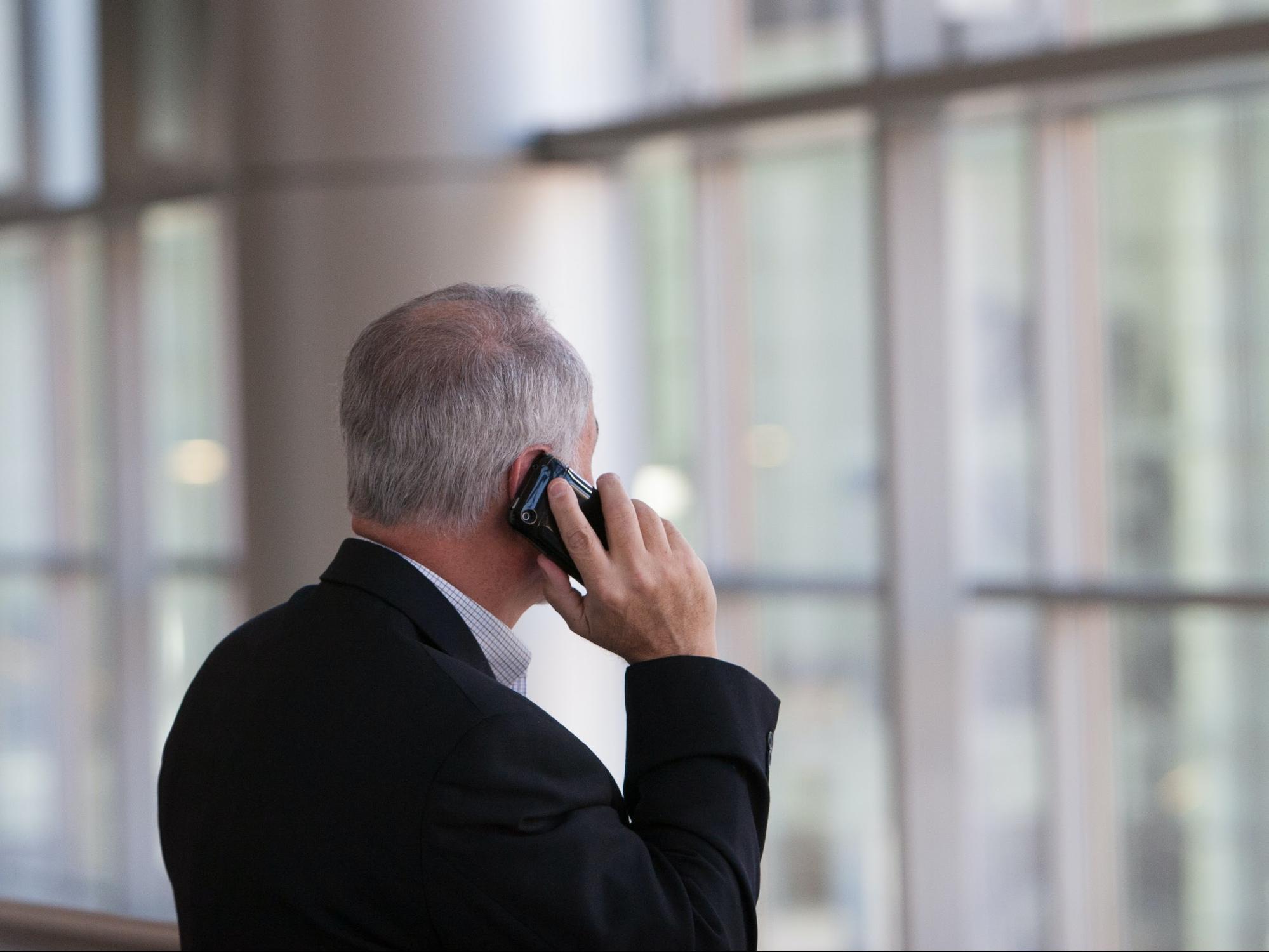
Telecom
Because the quantity of data being collected in the telecommunications space is so vast these days, the opportunities to translate these data into insights are immense. And while the analytical technology necessary to perform this work used to be highly sophisticated and inaccessible, recent improvements in computer hardware and software allow for users at all technical skill levels to gain something from their data. If you’re a professional in the telecom industry looking to venture into the world of data, you’ve come to the right place: this brief article covers some examples of how data can be utilized in the telecom space. We recognize telecom is a large field – these are just a few examples.
Business Intelligence Reporting
Business Intelligence (“BI” for short) refers to the technical process of collecting, storing, and analyzing the data that a business produces. In nearly all fields, from marketing to HR to sales to finance, even the most non-technical professionals are beginning to leverage BI to drive value for their businesses, and this trend extends quite well to the telecom space. There are several key components of competent BI practices:
Metrics
Knowing which metrics to track is half the battle when it comes to BI.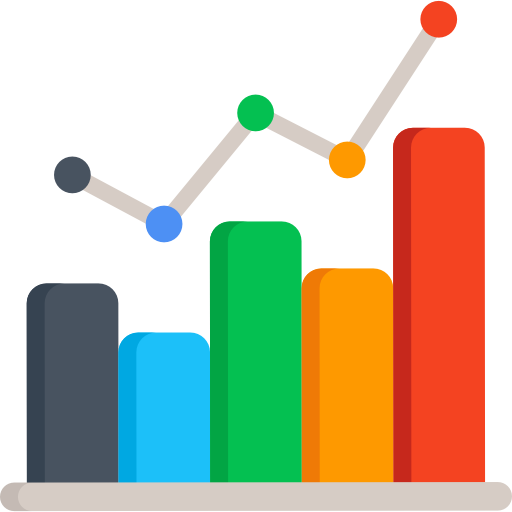
A marketing team may track click-through rates on their social media advertising to understand which advertisements are generating the most user interest; an HR analytics team may track turnover rates to gain insights on employee retention. Both of these are examples of BI analytics that a business may use. And when it comes to telecom, metrics to track depend on the segment of the telecom space in which your business operates. Popular metrics for a call center would include number of calls made and average time spent on calls; popular metrics for a telecom provider would include average revenue per user (ARPU), total number of subscribers, subscribers by geographical area, churn rates, and so on.
What really matters when selecting which metrics to track is to be answering the question(s) that your business wants answered; does your list of chosen metrics deliver you the insights that your business is looking to generate? If the answer to this question is yes, you are well on your way to a strong BI practice.
Software
Boxplot’s recommendation is to prefer PowerBI or Tableau, especially if your goal is to have your dashboards and other analytics update on a hands-free basis.
There are many options when it comes to which software to utilize for your organization’s BI, but the most popular choices would be Excel, PowerBI, and Tableau. Each of these three platforms has a massive user base and support community. The world’s leading BI specialists tend to prefer PowerBI and Tableau because of the visualization and real-time analytics options that each one has which are not available in Excel.
Fraud Detection
Unfortunately, phone scams are a real threat to consumers in today’s world. But modern algorithmic methods allow for telecommunications systems to automatically filter out fraudulent calls in real time. There are two main algorithmic techniques used to identify phone scams.
First, a data scientist can examine the data surrounding previous calls which were known to be fraudulent, and then use these data to effectively teach a computer how to identify and block future calls which are likely to be fraudulent as well; because data scientists can observe the accuracy of their algorithm before it is implemented in this case, we refer to this process as “supervised” machine learning.
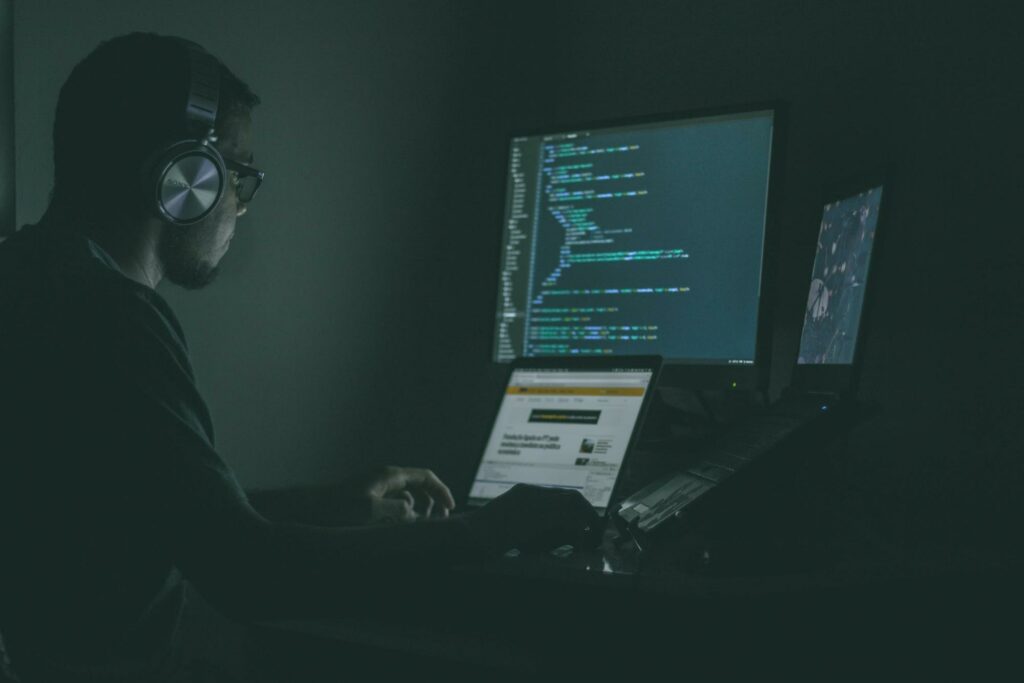
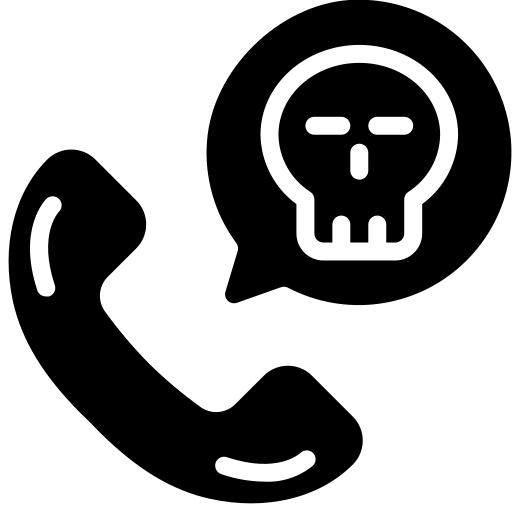
Second, a data scientist can identify statistical outliers from normally-observed patterns in call data. The idea being, clusters of extreme outliers from these data might suggest that something with the calls in those clusters is amiss. Although this can be a very effective method as well, we refer to this process as “unsupervised” learning because the data scientist does not differentiate between fraudulent and legitimate calls in the data they analyze, and thus cannot observe the accuracy of their algorithm prior to implementation.
Machine Learning: Recommendations
Call/text data and other phone usage information contains key insights about users’ preferences. As a result, data scientists can use supervised and/or unsupervised machine learning to accurately predict the products, services, and information in which those users are likely interested. This ability enables more targeted and effective advertising, marketing, and product recommendations to users across the network.
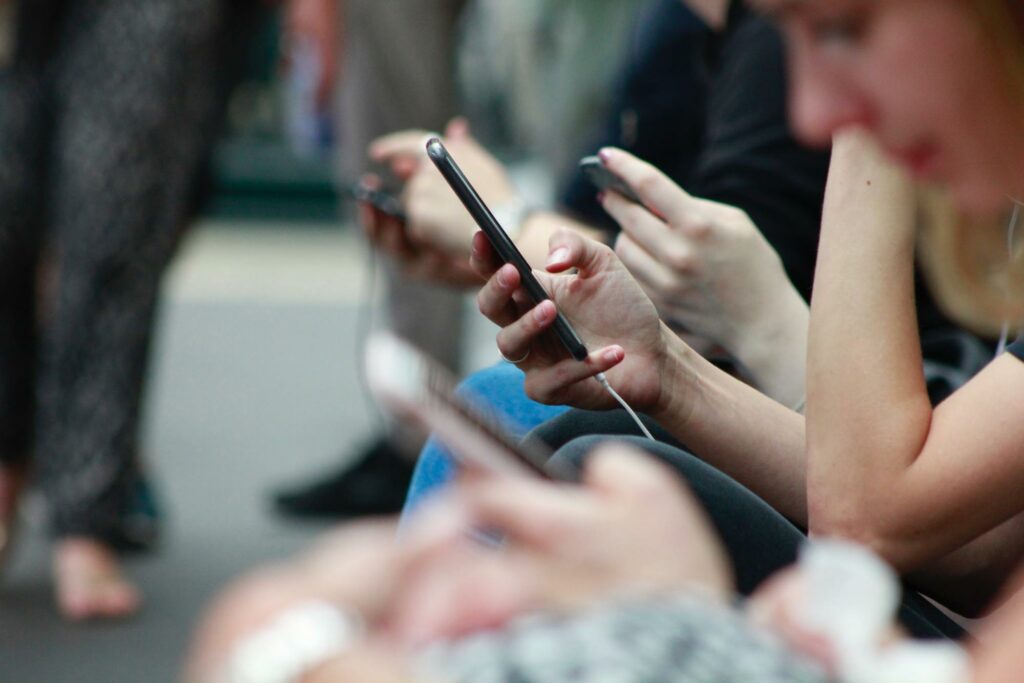
Machine Learning: Customer Churn Prediction
So you’ve created a robust customer inflow for your organization. Now comes the next challenge: how can you retain as many of our current customers as possible? Once again, the answer lies in your data. Implementing an effective machine learning algorithm will allow you to identify which clients are at risk of terminating their contracts, a month, a quarter, or even a year ahead of time; these insights then allow organizations like yours to take the proper interventions before it’s too late. As a result, you will be able to shift your intervention measures from reactive to proactive in a way none of your peer organizations can match.
Your Organization’s Data Strategy
Telecom firms across the industry are coming to recognize the benefits of a robust data strategy. Thus, if you’re in the telecom space, effective data science is your organization’s path to keeping pace with competitors. Whether your specific data-oriented goals are highlighted in this article or not, get in touch with us at Boxplot; we’re pleased to consult with professionals in the telecom industry, regardless of your prior level of experience with data. Contact us here, and start putting your data to work today.