AI Agents: The Good, the Bad, and the Unknown – Insights from Arthur’s Webinar
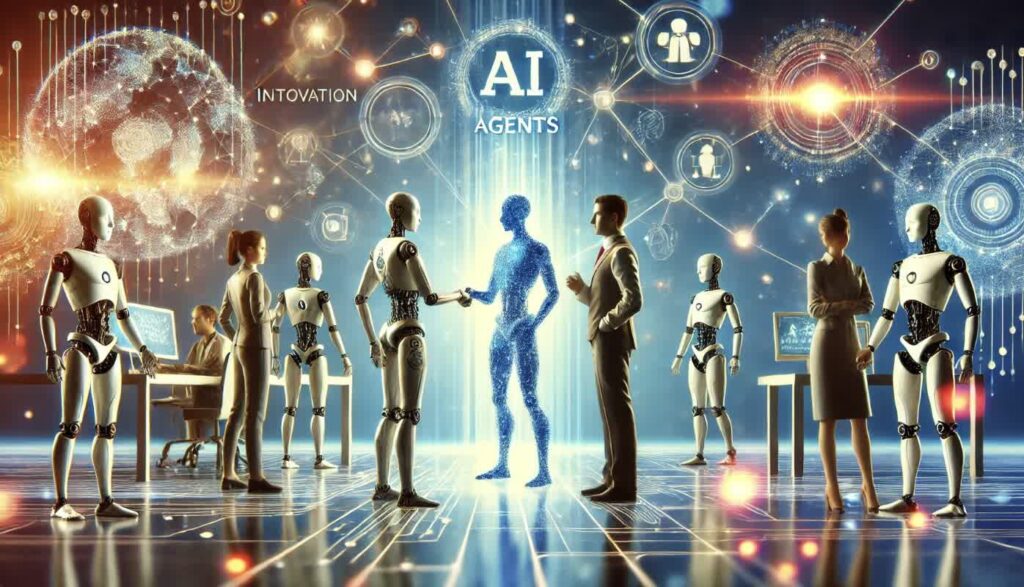
Artificial Intelligence is one of the fast-developing areas, and it is now all about the agents that are acting and redefining the interaction of humans with technology. From transforming industries to raising ethical questions, these agents are both promising and complex. Boxplot attended Arthur’s recent webinar, “A Quick Primer on Agents: The Good, the Bad, and the Future,” co-founder and Chief Scientist John Dickerson shared thoughts on the opportunities, challenges, and uncertainties of AI agents. Here’s a detailed recap of the key highlights.
The Good:
Enhancing Efficiency and Personalization
AI agents will continue making remarkable strides through increasing efficiency in user experiences, among others.
1. Simplification
AI agents make repetitive, time-consuming jobs easier. They free humans from monotony and menial resources to deal with much higher strategies. For instance, customer-care agents can multitask due to AI capabilities; thereby, many inquiries can be processed for quicker response and increasing customer satisfaction.
2. Tailored experience
AI agents are excellent at personalization, adapting to user preferences and behaviors to provide customized solutions. From personalized shopping recommendations to customized learning experiences in education, they are raising the bar on user experience standards.
3. Data-Driven Insights
Through the analysis of vast data, AI agents present actionable insights that help decision-makers act with more precision. This capability is transforming industries like healthcare, where agents assist in diagnostics and treatment planning.
The Bad:
Ethical Challenges and Risks
As AI agents become more prevalent, ethical considerations and operational risks demand attention.
1. Bias in Decision-Making
AI agents can perpetuate or even amplify biases present in their training data. These biases can lead to unfair outcomes in areas like hiring, lending, and law enforcement.
2. Privacy Concerns
To function effectively, AI agents often require access to sensitive data, raising questions about how this data is stored, shared, and secured. Without robust safeguards, user trust could be undermined.
3. Reduced Oversight
As AI agents handle more autonomous decision-making, there’s a risk of errors or unintended consequences. Establishing accountability and ensuring human oversight are critical challenges that must be addressed.
The Unknown:
Navigating Uncharted Territory
The future of AI agents is exciting but highly uncertain, with several questions defining the discourse.
1. Autonomous Decision-Making
As AI agents become more autonomous, how will we be able to align them with human values? A balance between independence and accountability has to be struck.
2. Regulation and Governance
The regulatory landscape for AI agents is still in its infancy. It will be important to develop clear guidelines and policies that balance innovation with ethics and safety.
3. Quantum Computing Impact
Quantum computing could increase the capabilities of AI agents exponentially. It may also introduce new risks, such as vulnerabilities in encryption. How will this technological leap shape the role of AI agents?
4. Collaboration with Humans
How can we design AI agents to augment human creativity, rather than displacing it? The construction of collaborative AI systems is one of the major tasks that will guarantee the constructive impact of AI.
Key Takeaways from the Webinar
Proactive Ethics: Early addressing of ethical challenges is a significant issue in making AI agents serve humanity responsibly.
This, in turn, requires that leaders of industry, policy, and research come together to provide a balanced framework for innovation in AI.
AI Literacy: Educating users about the capabilities and limitations of AI agents is crucial for gaining their trust and ensuring responsible engagement.
Agility: As AI evolves, the ability to adapt will be a defining factor in how well organizations can leverage its potential.
Conclusion:
We found Arthur’s webinar, “A Quick Primer on Agents: The Good, the Bad, and the Future,” to be a delightful discussion and comprehensive dive into AI agents. It communicated not just the potential of AI agents but also underlined responsible innovation that comes only by collaboration. As technology gets embedded in our day-to-day living, we must also deal with its many challenges and uncertainties if their benefits create significant value for good. This was a good introduction into AI Ethics as well.